Introduction
Smoking is known to cause various types of cancers, including lung cancer, laryngeal cancer, esophageal cancer, oral cancer, and pancreatic cancer, as well as chronic obstructive pulmonary disease, coronary artery disease, stroke, and circulatory system diseases (Centers for Disease Control and Prevention, 2020). In 2017, the overall rate of smokers over 19 was 22.3% in Korea, with 38.1% of men classified as smokers and only 6.0% of women (Cho, 2019). Although the smoking rate in Korea has steadily decreased over time, the finding that 31.6% of males over 15 years of age smoked in 2017 reflects a relatively high proportion compared to other Organization for Economic Co-operation and Development countries, as this was the second highest national smoking rate after Turkey (40.1%), followed by Japan (29.4%), France (25.8%), Spain (25.6%), Italy (25.1%), Germany (22.3%), the UK (19.1%), Mexico (12.0%), the USA (11.5%), and Sweden (10.5%) (Organization for Economic Co-operation and Development, 2019).
Information seeking, which has emerged as an important topic within health communication over the past few years, can be described as a deliberate effort to acquire information, either out of necessity or to fill gaps in one’s knowledge (Case, Andrews, Johnson, & Allard, 2005; Griffin, Dunwoody, & Neuwirth, 1999). When people lack knowledge of a disease, they will take certain steps to inform themselves about effective preventive measures, proper methods of early detection, and access to health care, which are important precautionary behaviors (Rimal & Real, 2003).
The intention of smokers to seek health information can also help individuals to quit smoking successfully. For example, Killian (2012) showed that individuals who search for health-related information on the internet were less likely to be smokers. Van Der Rijt and Westerik (2004) also showed that smokers seeking information about smoking cessation were more willing to participate in a smoking cessation program.
However, despite the distinct advantages of seeking information, people are not always motivated to do so on their own. In particular, people often avoid seeking information when they find it distressing (Brashers et al., 2000; Leydon et al., 2000) or when it differs from their own beliefs with which they are comfortable (Babrow, 2001). Therefore, this study investigated the antecedents of information seeking, as a self-protective behavior to improve the health outcomes of smokers, and aimed to confirm their influence.
Theoretical Framework
Protection motivation theory (Rogers, 1975, 1983) and the health belief model (Janz & Becker, 1984; Rosenstock, 1974; Sheeran & Abraham, 1996) are representative health communication theories, according to which perceived risk is a main factor for predicting health outcomes. In contrast, the risk perception attitude (RPA) framework argues that predictions of health outcomes must take into account efficacy as well as risk perception (Rimal, 2001).
The RPA framework is based on the extended parallel process model (EPPM) (Rimal, 2001; Rimal & Real, 2003), which combines the parallel process model (Leventhal, 1970, 1971) with protection motivation theory (Rogers, 1975, 1983) to compensate for the theoretical limitations of risk perception and its inconsistent relationship with health outcomes.
In the EPPM model, people with a high threat perception are divided into two different behavior pathways (the danger control process and the fear control process) and exhibit response behaviors to diseases in order to control the threat. Efficacy beliefs are thought to shape different behavioral pathways, which correspondingly lead to different health behavior outcomes for diseases. The danger control process leads to positive health behavior, while the fear control process leads to behavior that avoids or rejects health behavior. Perceived threats motivate a behavioral response, but at the same time, they can trigger a feeling of fear. If one’s efficacy is low, fear is amplified, prompting a defensive motivation for controlling fear; thus, the fear control process is undertaken to avoid or reject the threatening situation. On the contrary, high levels of efficacy weaken one’s sense of fear and prompt the danger control process, which enables one to overcome the perceived threat, leading to health behaviors that can prevent disease.
The RPA framework follows the EPPM model, which sees efficacy beliefs as a moderating variable between risk perception and health outcomes. Witte (1992, 1994) emphasized that preventive behaviors to protect an individual’s health from a disease are not stimulated by perceived risk and that efficacy beliefs are essential for preventing disease. In other words, while perceived risk motivates preventive behaviors to escape the threat of disease, the actual preventive measures to respond to the threat of disease are more directly controlled by an individual’s efficacy beliefs (Witte, 1992, 1994).
As such, the RPA framework based on the EPPM model conceptualizes risk perception as a motivator to avoid diseases and efficacy beliefs as facilitators of behavioral change. There are four possible behavior classifications depending on risk perception and efficacy beliefs: responsive (high risk perception, high efficacy), avoidant (high risk perception, low efficacy), proactive (low risk perception, high efficacy), and indifferent (low risk perception, low efficacy).
Responsive behavior is shown by people with a high level of risk awareness about smoking and a high level of belief in their knowledge and ability to prevent smoking-related diseases. Such individuals are likely to respond immediately to new threats and take precautions to protect themselves from smoking-related illnesses and minimize damage.
Avoidant behavior occurs in people with a high level of awareness of the risks of smoking, but a low level of belief in their knowledge and ability to prevent smoking-related diseases. Such individuals take the risk of smoking-related illness into consideration, but fail to take corresponding measures to reduce their risk, or intend to avoid the risky situation altogether.
Proactive behavior is shown by people who are not well motivated, due to their low level of risk perception, but are likely to take measures before developing smoking-related diseases due to their high level of belief in their knowledge and ability to prevent smoking-related diseases.
Finally, indifferent behavior occurs in people with low levels of both risk perceptions and efficacy beliefs. In other words, they have no interest in the damage caused by smoking, little knowledge of smoking-related illnesses, and a low ability to avoid dangerous behaviors.
Corcoran (2013) and numerous other researchers (Cummings, Jette, Brock, & Haefner, 1979) have pointed out that theories that do not account for social norms and social and psychological factors are limited in their ability to predict health outcomes. Furthermore, according to Bettenhausen and Murnighan (1985), norms are some of the least visible aspects of human behavior, but function as a powerful form of social control. For this reason, the influence of norms when predicting an individual’s health outcomes should not be overlooked.
According to the theory of planned behavior, health behaviors are influenced not only by cost-benefit analyses and rational decision-making, but also by perceived behavioral norms within a reference group. Therefore, norms provide a social context and exert a strong influence on individuals’ decision-making process concerning their health behavior (Sorensen, Emmons, Stoddard, Linnan, & Avrunin, 2002).
Individuals who feel that they have normative support for a particular issue or behavior from a reference group are more likely to act in a way that is consistent with their attitudes than those who are not (Terry & Hogg, 1996; Terry, Hogg, & White, 1999). Numerous studies on the drinking behavior of adolescents show that the influence of a reference group acts as an important variable in individuals’ drinking behaviors (Kuther, 2002). The drinking behavior of adolescents is guided by the perceived norms of others around them, rather than by a cost-benefit analysis based on rational decision-making.
The theory of planned behavior (Ajzen, 1991) views subjective norms as a predictor of health-related behavioral intention, along with attitudes toward behavioral control and health-related behavior itself. However, several studies dealing with the relationship between norms and health outcomes have argued that norms not only act as predictors of health-related behavioral intention, but also as mediators or moderators. The main impact of norms is to directly affect health-related behavioral intentions, but norms also have the effect of mediating or moderating the relationship between perceived risk, efficacy, and health-related behavioral intention (Cummings et al., 1979; Park & Smith, 2007).
With the increasing tendency to treat patients as active consumers of healthcare (Frosch & Kaplan, 1999), information seeking has become a key concept in health communication. Health-related information seeking can lead to a deeper understanding of disease symptoms, prevention tactics, and effective cures by reducing uncertainty (Turner, Rimal, Morrison, & Kim, 2006). Seeking health information has been proven to be related to various health outcomes such as changes in cognition, behavior, and affect (Lambert & Loiselle, 2007). More specifically, depending on a person’s judgments, beliefs, and attitudes, seeking health information can have an impact on decisions to undertake health-promoting behaviors (Lambert & Loiselle, 2007; Upadhyay, Lord, & Gakh, 2019).
In particular, seeking health information can provide motivation for more informed decisions and actions to maximize the positive effect of health behaviors (Anker, Reinhart, & Feeley, 2011; Ramirez et al., 2013). Health information seeking can include internet searches, discussions with healthcare providers, and hotline calls regarding health-related topics (Anker et al., 2011). Low interest in health information seeking has been associated with negative health outcomes, including delayed diagnosis or treatment of diseases and persistent unhealthy behaviors (Johnson, 2014).
Moreover, according to large health campaigns like the Stanford Five-City Project (Winkleby, Flora & Kraemer, 1994) and the Minnesota Heart Health Program (Viswanath & Finnegan, 1996), information seeking and retention of knowledge can lead to positive health outcomes. It also has been proven that the positive effects of these large health campaigns continue to be effective and stimulate information-seeking behavior even after the campaign ends (Rimal, Flora, & Schooler, 1999). Therefore, information seeking can be considered an important factor for self-protective behavior (Rimal & Real, 2003).
The research model presented in Figure 1 empirically examined the effect of risk perception, efficacy beliefs, and subjective norms on information seeking and the moderating effect of subjective norms on information seeking within the RPA framework.
The RPA framework conceptualizes efficacy beliefs as a moderating variable to explain the lack of consistent support for the causal relationship between risk perception and self-protective motivations and behaviors in health communication research (Rimal & Real, 2003). However, tests of the RPA framework have produced inconsistent findings; specifically, survey-based research (Rimal, 2001; Rimal & Real, 2003; Rimal, Böse, Brown, Mkandawire, & Folda, 2009) has reported more theory-consistent results than experiment-based research (Rimal & Real, 2003; Turner et al., 2006; Zhao & Cai, 2009). From these findings, it is reasonable to infer that risk perception or efficacy beliefs can play a major role in the RPA framework. In addition, it has been widely reported that subjective norms, which account for individuals’ awareness about the beliefs and actions of others (Fishbein, 1980), have been considered as a main factor for improving predictions about the results of health-related behavioral intentions (Corcoran, 2013; Cummings et al., 1979). Thus, self-protective motivations and behavioral intentions can be affected by subjective norms as well as by rational decision-making stemming from individual risk perception and efficacy beliefs. Thus, the following hypotheses are posited:
-
Hypothesis 1: Risk perception is positively associated with information seeking.
-
Hypothesis 2: Efficacy beliefs are positively associated with information seeking.
-
Hypothesis 3: Subjective norms are positively associated with information seeking.
In some studies, subjective norms showed direct and moderating effects between perceived risk, efficacy beliefs, and health-related behavioral intentions (Cummings et al., 1979; Park & Smith, 2007; Park, Klein, Smith, & Martell, 2009). Thus, the following hypotheses are posited:
Methods
In the RPA framework, risk perception consists of severity and susceptibility. Severity refers to the degree to which one perceives a disease as serious, and susceptibility indicates the degree to which one perceives himself or herself to be susceptible to a disease. Efficacy beliefs consist of response efficacy, which is one’s belief in the effectiveness of health behaviors for preventing diseases which may pose a threat, and self-efficacy, which considers one’s capability to perform preventive health behaviors.
Variables used in the study model were developed to be measured using a 5-point Likert scale. To ensure the face validity of each variable’s measurement items, the measurement items from existing theories and prior studies were derived, with appropriate modifications for application to smokers. Operational definitions and related studies of the variables are shown in Table 1.
Variable | Operational definition | Reference | |
---|---|---|---|
Risk perception | Severity | The seriousness of the consequences of the health problem. | Rimal et al. (2009); Turner et al. (2006) |
Susceptibility | The likelihood that one would experience a certain health problem. | ||
Efficacy beliefs | Self-efficacy | The extent to which people believe they are capable of performing specific behaviors to attain certain goals. | Rimal and Real (2003); Turner et al. (2006) |
Response efficacy | The extent to which people believe that a recommended response is effective at deterring or alleviating a health threat. | ||
Subjective norms | Individuals’ awareness about the beliefs and action of others. | Fishbein and Ajzen (1977); Greene, Hale, and Rubin (1997) |
|
Information seeking | Intention to seek information, behavioral intention, and knowledge acquisition. | Rimal and Real (2003) |
In order to verify the impact of risk perception, efficacy beliefs, and subjective norms on health-related information seeking, data were collected through a survey of undergraduate and MBA students majoring in business administration at a large university in Seoul, Korea. To fulfill the purpose of the study, respondents were limited to current smokers who had smoked at least 100 cigarettes in his or her lifetime, as defined by the United States Centers for Disease Control and Prevention. Of the 350 survey responses collected, 311 responses were used for analysis after excluding 39 unreliable responses. The demographic data of the respondents are shown in Table 2.
Results
This study used SPSS version 26 and SmartPLS 2.0 to analyze the data. SPSS 26 was used to calculate Cronbach’s alpha values to verify the reliability of the measurement items, and SmartPLS 2.0 was used for confirmatory factor analysis (CFA) and hypothesis testing through structural equation modeling.
Before verifying the structural model, the reliability and validity of the measurement model were verified by conducting CFA using SmartPLS 2.0 to confirm whether the questionnaire items were configured and measured according to the research purpose. Since the model contains second-order variables, the factor scores of the first-order constructs were used to create second-order constructs (Chin, Marcolin, & Newsted, 2003). Following the suggestion of Thornton, Henneberg, and Naudé (2014), the validity of second-order variables was examined at the level of the first-order constructs.
With regard to reliability, the composite reliability (CR) value and the Cronbach’s alpha value were measured, both of which are considered to indicate high reliability if the value is greater than 0.7 (Nunnally, 1975) and satisfactory reliability if the value is greater than 0.6 (Hair, Sarstedt, Pieper, & Ringle, 2012). As shown in Table 3, the Cronbach’s alpha and CR values were 0.661 and 0.803, respectively, confirming reliability.
Next, in the analysis of average variance extracted (AVE), all variables exceeded the standard value of 0.5 (Fornell & Larcker, 1981) and all factor loadings were found to be significant (with values greater than the standard value of 0.5), indicating convergent validity (Bagozzi, Yi, & Phillips, 1991), with the exception of SELF1 and SELF2, which were eliminated since they had values below 0.5.
Additionally, the discriminant validity was verified by determining whether the square root of the AVE of each construct was higher than the correlation between that construct and the other constructs. Table 4 demonstrates that the square roots of the AVE for constructs shown in bold italics were higher than the correlation between those constructs and the others, thus confirming the discriminant validity of the measurement model (Fornell & Larcker, 1981).
Construct | RES | INFO | SELF | SEV | SUB | SUS |
---|---|---|---|---|---|---|
RES | 0.713 | |||||
INFO | 0.434 | 0.835 | ||||
SELF | 0.152 | 0.206 | 0.765 | |||
SEV | 0.328 | 0.236 | 0.141 | 0.797 | ||
SUB | 0.371 | 0.369 | 0.307 | 0.156 | 0.738 | |
SUS | 0.384 | 0.274 | 0.107 | 0.572 | 0.203 | 0.820 |
In this study, the partial least squares structural equation modelling (PLS-SEM) method was used to verify the hypotheses, and SmartPLS 2.0 was used as an analysis tool. PLS-SEM is recommended for when the number of samples is small, when the data do not follow a normal distribution, when the rationale is not clear, or when second-order variables are used. In this study, since risk perception and efficacy were measured as second-order variables, it was judged appropriate to use the PLS structural equation model rather than a covariance-based structural equation model employed in AMOS and LISREL.
The structural model was evaluated using the variance of the described endogenous variables (R2) and the values of the path coefficients (β) that passed the significance level test. As second-order variables, risk perception and efficacy beliefs are formed using factor scores of the first-order constructs of severity, susceptibility, self-efficacy, and response efficacy (Bock, Zmud, Kim, & Lee, 2005). A bootstrapping resampling method (311 samples, 5,000 repetitions) was performed, and three hypotheses (hypotheses 1, 2, and 3) were supported as shown in Figure 2, showing the paths’ coefficients and t-statistic values for the structural models. Both risk perception and efficacy beliefs, which comprise the RPA framework, were positively associated with information seeking (β = 0.114, p < .05 and β = 0.305, p < .001, respectively), thus supporting hypotheses 1 and 2. Subjective norms are also significantly associated with information seeking (β = 0.213, p < .001), supporting hypothesis 3. Risk perception, efficacy beliefs, and subjective norms explained approximately 25% of variance in information seeking (R2 = 0.247).
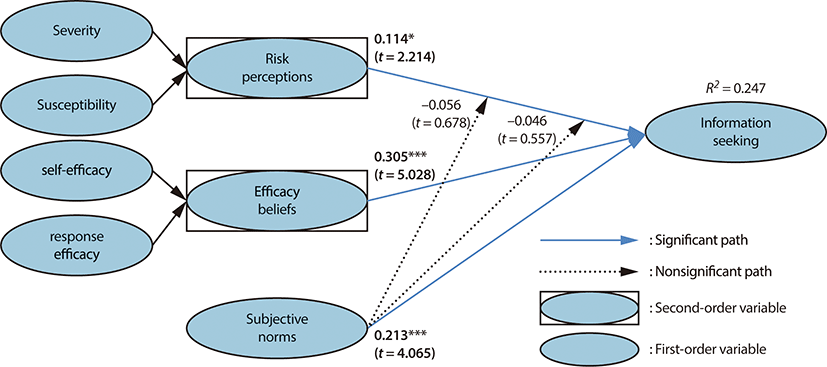
A further analysis was conducted to explore whether subjective norms moderated the effect of risk perception and efficacy beliefs on information seeking. No moderating effect was found, and hypotheses 4a and 4b were therefore rejected. The results of the moderating effect analysis are shown in Table 5.
Hypothesis | Path | Standardized β | t-value | Result |
---|---|---|---|---|
H4a | Risk perception → Information seeking | 0.557 | –0.046 | Not supported |
H4b | Efficacy beliefs → Information seeking | 0.678 | –0.056 | Not supported |
Discussion
This study verified the effectiveness of the two components of the RPA framework (risk perception and efficacy beliefs, which represent risk perception attitudes toward smoking by smokers) and subjective norms, which show normative support for a particular issue or action from a reference group, on health-related information seeking. Further verification of the effectiveness of the RPA framework on information seeking was conducted by investigating the potential moderating effect of subjective norms.
First, risk perception and efficacy beliefs were confirmed to have a significant positive effect on information seeking, confirming hypotheses 1 and 2. This result is consistent with the findings of previous studies (Rimal, 2001; Rimal & Real, 2003; Rimal et al., 2009), which have shown that risk perception and efficacy beliefs play important functions in one’s intention to seek health-related information. While most existing studies (Rimal, 2001; Rimal et al., 2009; Zhao & Cai, 2009) examined the interaction between risk perception and efficacy beliefs, this study also examined and compared the magnitude of their effects, and found that efficacy beliefs had a stronger effect than risk perception. Therefore, efforts to improve information seeking should preferably focus on increasing the effectiveness of preventive health behaviors and one’s ability to undertake preventive behaviors, rather than on raising awareness of the risks of smoking and smoking-related diseases.
Second, it was confirmed that subjective norms also had a significant positive effect on information seeking (hypothesis 3), and their influence was found to be stronger than that of risk perception. While studies on organ donation (Park & Smith, 2007) and alcohol consumption (Park et al., 2009) showed that subjective norms had a greater impact as indicators of participation than efficacy beliefs, this study demonstrated that subjective norms had a weaker effect on information seeking than efficacy beliefs, but a stronger effect than risk perception. Based on these results, it can be inferred that subjective norms should be taken into account when predicting health-related behavioral intention related to smoking, although some variation may be present across different diseases and risk behaviors.
Lastly, subjective norms did not moderate the effects of risk perception and efficacy beliefs, as variables in the RPA framework, on information seeking. As proposed in hypothesis 3, subjective norms, which represent perceptions of other people’s beliefs and behaviors regarding smoking, are influential enough to have a main effect, rather than exerting a moderating effect. This suggests that smokers are directly affected by negative perceptions from a reference group, such as the effect of stigma on smoking behaviors and secondary damage caused by second-hand smoke.
The main limitation of this study relates to the representativeness of its sample. Since the survey was conducted exclusively among university students and MBA students at a large university in Seoul, the results of this study should not be generalized. Therefore, future studies should use more systematic sampling methods to collect data from various groups. Another limitation of this study as it relates to the RPA framework is that it was not possible to analyze responses according to whether participants displayed responsive, avoidant, proactive, or indifferent behavior due to difficulties stemming from the sample size. Future studies should embark upon such an analysis.
Conclusion
The intention of smokers to seek health-related information is considered a self-protective behavior and can be the first step for taking preventive measures to avoid smoking-related diseases such as lung cancer or quitting smoking altogether. In this study, we found that three variables—risk perception, efficacy beliefs, and subjective norms—had positive impacts on smokers’ intention to seek health-related information. The variable with the greatest overall effect was efficacy belief, followed in order by subjective norms and risk perception. Thus, in order to encourage smokers to seek health-related information in anti-smoking campaigns, it is important to improve their perceptions of efficacy and find ways to shape their norms by incorporating input from an influential reference group, rather than merely highlighting the health risks of smoking.